- | Technology and Innovation Technology and Innovation
- | Policy Briefs Policy Briefs
- |
The Future of Materials Science: AI, Automation, and Policy Strategies
Three objectives for federal policymakers to advance the pace and quality of materials discoveries
The limits of technology expand the limits of human capabilities. The technology humans can create is fundamentally constrained by the materials used to build that technology. Nearly every technological epoch in human history has been enabled by breakthroughs in materials. Bronze, iron, plutonium, and, most recently, silicon all undergird novel ways of enriching—as well as destroying—human livelihood.
Johannes Gutenberg’s alloy of lead, tin, and antimony became the basis of movable type—the printing press—which, in turn, heralded the first information revolution in the 15th century. Moveable type was the seed of modern civilization and the starting point of ideological, religious, and political turmoil. A few centuries later, other advancements in metallurgy (themselves spurred on by the more rapid dissemination of information enabled by the printing press) allowed for the invention of weight-driven clocks far more accurate than the water-driven clocks then in use.[1] Time itself, suddenly, could be measured scientifically.
Isaac Newton would go on to use these weight-driven clocks for his experiments that shattered two thousand years of scientific dogma and birthed the Newtonian view of nature and human affairs. Newton would not have deduced that gravity pulls objects toward the Earth at 9.8 meters per second with a water clock, after all. With weight-driven clocks, supervisors of the nascent 18th-century industrial enterprises could suddenly measure labor productivity, thereby laying the groundwork for the impending era of industrial capitalism.[2]
Untold possibilities await within the discipline that today we call “materials science”—the exploration of novel ways to configure matter to accomplish useful things. The macrohistory of this discipline mirrors that of many other domains of science—low-hanging fruit, plucked here and there, almost as if by chance—until around the late 18th and early 19th centuries, after which the pace of discovery picked up dramatically. All this was sustained until the mid-20th century—but then came the so-called Great Stagnation, a period of reduced productivity, across both science and the economy, that has persisted for the past half-century.
Today, materials science finds itself in an all-too-familiar predicament. The low-hanging fruit has seemingly all been plucked; so, too, has the mid-hanging fruit. Discovery is getting harder, and, so, the methods by which discoveries are made must be reimagined.
Artificial intelligence (AI) shows great promise as a foundation for that reimagining. Yet too often, AI is bandied about as a panacea, offering near-magical computational insight. The reality is far from that simple. AI, for science, is, paradoxically, both over- and underrated. It is overrated as a near-term “miracle machine,” yet it is underrated in its long-term potential.
AI should be understood, in this context, as a foundation for the reimagining of materials science as an enterprise. But a foundation alone does little, just as the foundation of a home is not equivalent to four walls and a roof. Indeed, a new foundation requires new structures to be built upon it. Similarly, to maximize the potential of AI, materials science will need to adopt new practices, methodologies, assumptions, and aspirations. The academic, corporate, governmental, and philanthropic funders of materials science will need to do the same to take advantage of new opportunities presented by AI. AI allows scientists to generate far more ideas for breakthroughs, but in order to achieve those breakthroughs, scientists’ ability to test those ideas in experiments will need to scale along with AI. Materials science will need to transition from an artisanal scale to an industrial scale. The practices, equipment, and labs of materials science will all need to be transformed to reflect this new reality.
Nothing about this transformation will be easy, and none of it will happen overnight. This report will focus on what public policy and research can do to accelerate the transition from artisanal- to industrial-scale science. It will begin with a brief overview of materials science as a modern scientific enterprise and will attempt, in particular, to illustrate the enormous economic and technological potential of the field. It will then examine the promise of AI in advancing materials science as well as the limitations of existing applications of AI to the practice of materials science. Then, it will present an agenda for public policy and public scientific research funding—an agenda that will allow materials discovery and testing on a far greater scale than is possible today. The agenda is focused on five priorities:
- Articulating the role of the Department of Energy (DOE), the National Science Foundation (NSF), and other agencies
- Maximizing the utility of preexisting public datasets relevant to materials science
- Training materials science foundation models
- Researching and constructing robotic cloud laboratories to enhance experimental throughput
The Promise of AI and Materials Science
What is materials science?
Materials science is the discipline that investigates “the relationships that exist between the structures and properties of materials.”[3] Materials engineering, by contrast, involves using the discoveries of materials scientists to create novel products, processes, and other practical innovations. This paper focuses principally on materials science in the hope that materials engineers will have a far larger toolkit in the decades to come.
Almost every part of the built environment of advanced capitalist societies is downstream of an advancement in materials science—and often not one, but dozens, hundreds, or thousands of separate advancements. Materials science encompasses a vast range of subfields and related scientific domains.
New materials can be used to make better battery anodes and cathodes, enabling longer-lasting, faster-charging, safer, and more reliable laptops, electric vehicles, and smartphones—and, over the long term, a more resilient electrical grid through utility-scale energy storage. New kinds of magnets are bringing innovations like commercially viable nuclear fusion closer to reality, inch by inch. For advances in semiconductor speed and efficiency to continue, materials science will play a starring role. Moore’s Law, after all, is not a law of nature—it is a law that humans enforce, and we do so through ingenuity. Currently, nearly every mass-produced good relies upon a bevy of industrial materials: lubricants, adhesives, bindings, coatings, catalysts, and more. Each of these materials has its own sizable subfield of materials science.
What unites this staggeringly diverse range of intellectual inquiries is a process: Materials science is a search over a near-infinitely vast landscape of potential materials. There are more ways to assemble matter than there are atoms in the universe. Thus, it is impossible to take the naïve approach of “trying everything.” Prioritization is essential, informed by knowledge, heuristics, intuitions, and tools that enable scientists to refine their search. It is precisely here that AI holds the greatest promise, yet the exact nature of this promise is often misunderstood.
The role of AI in science
Popular media often imagines AI as a kind of genie, furnishing superhuman insights on a grand scale. AI is often given the honor of being the subject of a sentence: “AI does this,” “AI invents that.” This even occurs in materials science. A 2023 blog post from Google DeepMind, summarizing the company’s GNoME model for materials science (more on that topic below), said that the model’s “discovery of 2.2 million materials would be equivalent to about 800 years’ worth of knowledge.”[4]
Perhaps one day. But for now, the reality is more complex.
First, it is worth disentangling competing definitions of AI. In contemporary vernacular, AI is often used almost metonymically to refer to large language models like OpenAI’s ChatGPT, Anthropic’s Claude, or Meta’s Llama. These generally useful models are research artifacts released by their creators on the path toward “artificial general intelligence,” (AGI) a loosely defined concept referring to AI systems whose intelligence in all domains matches that of analogous human experts. Such an invention would indeed have profound effects on all science, and some—including the companies themselves—believe it is imminent. Yet because it remains speculative and under specified, AGI will not be a focus of this study. Language models will figure slightly, predominantly in the section focused on automated laboratories.
Instead, this paper discusses AI in reference to deep neural networks trained on enormous volumes of data with large amounts of computational power. The above-mentioned language models certainly are examples of this, but the field is much larger than just large language models. Specifically, this paper focuses on deep neural networks trained on huge quantities of scientific data—in our case, data relating to the structure, properties, and behavior of materials. Examples of recent foundation models in materials science include DeepMind’s GNoME and Microsoft’s MatterGen, as discussed below.
AI models of this kind have already been used to great effect in a diverse range of scientific fields—perhaps most notably in biology. Google DeepMind’s AlphaFold, which debuted in 2019, predicted— with better accuracy than any other computational method at the time—what protein structures would be made from specific amino acid sequences.[5] AlphaFold 2, released in 2021, achieved significantly higher levels of accuracy than the original.[6] And AlphaFold 3, released in 2024, has the expanded ability to model the interactions between proteins and other biomolecular compounds and predict the structure that results when proteins bind with other biomolecules.[7] In other domains of biology, deep neural networks have been used in combination with genomic data (nucleic acid sequences) to predict gene sequences and even the full genomes of novel forms of prokaryotic (single-celled) life.[8] These are just a few of the ways in which deep neural networks are being applied in biology.
Though the specifics differ, deep neural networks are all predictive models—that is, they are fed a sequence of input data, perform mathematical operations to transform that data, and output a related prediction, such as the structure of a protein, the way biomolecules will interact, or the genetic sequence of a species. The mathematical operations performed on the input data are primarily learned through the training process, which involves the model making predictions on input data where the correct output data is known. For protein structures, this would be, for example, proteins whose amino acid sequences and resultant three-dimensional structures are both established.
Critics of deep learning (the use of deep neural networks) have argued that deep neural network models are simply “pattern matching,” and that they will struggle to generate valid results when operating “out of distribution,” or in domains with no verified data on which to train.
By and large, these criticisms underrate the nature of the “pattern matching” that happens within a well-trained deep neural network. It is well understood by now that large language models learn high-order concepts and abstractions, and recent advancements in “mechanistic interpretability” have proven that contemporary language models have a rich understanding of an enormous range of human-interpretable concepts.[9] It has even been suggested that modern language models pick up on structures in language that humans do not readily understand.[10] Indeed, the ability of neural networks to learn the underlying structure of their training data has been theorized since the early days of the field.[11]
When mechanistic interpretability techniques are applied to biological foundation models, they reveal that these models, too, pick up on high-order abstractions in biology. In other words, despite not being “told” about high-order biological concepts that humans have discovered through centuries of experimentation, deep neural networks were able to discover them simply from the raw data.[12] And, just as researchers in natural language processing suspect that current language models may understand even higher-order concepts for which humans have only a partial vocabulary, developers of a recent protein model called ESM3 suggested the same may be true in biology—specifically, that deep neural networks may be learning to simulate evolution:
We have found that language models can reach a design space of proteins that is distant from the space explored by natural evolution, and generate functional proteins that would take evolution hundreds of millions of years to discover. Protein language models do not explicitly work within the physical constraints of evolution, but instead can implicitly construct a model of the multitude of potential paths evolution could have followed. . . .
ESM3 is an emergent simulator that has been learned from solving a token prediction task on data generated by evolution. It has been theorized that neural networks discover the underlying structure of the data they are trained to predict. In this way, solving the token prediction task would require the model to learn the deep structure that determines which steps evolution can take, i.e. the fundamental biology of proteins.[13]
Neural networks are not mere stochastic parrots, uselessly mimicking their training data. Rather, they learn salient concepts in their underlying training data, and that implies that their utility to all domains of science—materials science included—will be significant. Yet neural networks are not oracles; despite AlphaFold having existed in increasingly advanced forms for half a decade, it and other models are not magically generating perfectly valid medicines. Rather, AlphaFold’s predictions—even sometimes ones the model gives with high confidence—are often flawed.[14] AlphaFold and other biological foundation models are tools that scientists use to refine their search for new therapeutics or other useful biomolecules. Instead of being used to circumvent costly and time-consuming experiments, deep learning models can guide scientists toward the most productive possible experiments.
Deep learning will likely continue guiding scientists toward experiments in materials science for the foreseeable future.
AI and materials science
With both the potential and the limitations of deep learning in mind, we will now look at the existing applications of AI to the field of materials science. While this is a nascent field compared to biology, a great deal of work has already been done. In the past two years, large corporations such as Microsoft[15]and Meta,[16] as well as academia[17]have made significant progress in applying deep learning techniques to materials science research.
One of the recent prominent contributions to this field has already been mentioned: Google DeepMind’s graph networks for materials exploration (GNoME).[18] GNoME exemplifies both the promise and the pitfalls of deep learning in materials science. A basic description of the approach taken by Google DeepMind and the critical reaction of some in the materials science community will, therefore, be instructive.
At its core, the GNoME project uses graph neural networks—a form of deep neural networks used to represent entities (nodes in the graph) and the relationship between the entities (these are referred to as “edges” and can be thought of as lines connecting the nodes). GNoME represents each atom in a compound as a node in the graph and encodes the distances between atoms as the edges. In this way, the network is trained on not just raw sequences of atoms in a compound but instead the structure of that compound.
The core graph neural network in the GNoME project predicts the energy of a crystal structure. Lower-energy crystals are more likely to be stable in real life, so, by finding the low-energy configurations of a given set of atoms, the model is (in theory) predicting compounds that are likely to exist and be useful in the real world. This model does not, on its own, predict new material structures. Instead, it predicts the energy of the structures it is fed.
The researchers at DeepMind used a variety of methods for generating new potential materials (crystal structures) and fed those ideas into the model to form a dataset of crystals with predicted low energies. Crystals with predicted low energies were “repeatedly” validated using density functional theory (DFT), a computationally expensive method of validating molecular compounds that is based on formal physics rather than neural networks.[19] This is the source of the headline result of the paper: DeepMind’s GNoME system discovered 2.2 million “new crystals” and 380,000 “stable crystals.” DeepMind researchers took their claim even further when they asserted in a blog post that they had discovered “millions of new materials.”
Materials scientists have criticized GNoME and other applications of AI to materials science.[20] Scientists Anthony Cheetham and Ram Seshadri point out that the data on predicted stable crystals from GNoME are often organized in a way not useful to scientists, and that the data include a large number of radioactive materials “that are unlikely to have any utility in the materials world.” This flaw, perhaps, can be attributed to the deep learning ethos that undergirds DeepMind: Take as much data as possible, input that data into a neural network, and trust that deep learning will work. It must be said that while this approach has worked spectacularly well over the past decade in other situations, it occasionally results in the inclusion of low-quality training data.
More troublingly, Cheetham and Seshadri suggest that a large number of the predicted compounds are trivial variations on already-known materials. As the authors write, “We have yet to find any strikingly novel compounds in the GNoME. . . listings.” They instead suggest that, rather than “discovering millions of new materials,” the GNoME paper (and, by extension, other AI-based approaches to materials science) is “a list of proposed compounds.” In their view, a compound is a proposed configuration of matter, while a material is a configuration of matter that has been empirically shown to have utility. Just as with AlphaFold, a computational prediction (made using AI or other methods) is not, in itself, a new discovery. It is, if used well, a guide to refining a material scientist’s search through vast possibilities.
To summarize, the application of AI in biology should give us strong theoretical and empirical reasons for believing it can be productively applied to materials science. Existing uses of AI in materials science have been intriguing but have yet to live up to the hype or the potential. We have strong evidence to believe, based on the widely observed neural network “scaling laws,” that larger materials science foundation models, trained on more data and with more computing power will yield significant performance improvements. But in all domains of science, experimental validation of computational predictions will remain key for at least the foreseeable future, if not indefinitely. No matter how capable the neural network, the world is simply too complex to simulate computationally, and if we are going to use novel materials to build new aircraft, energy-generation equipment, and other critical technology, we will need to confirm the properties of those materials using real-world experiments.
Thus, larger neural networks alone will not allow materials science to grow to industrial-scale discovery and synthesis of novel compounds. This growth also requires a way to greatly expand “experimental throughput,” or the number of experiments a given scientist can perform over a period of time. This core intuition forms the basis of the policy agenda to be discussed.
Why Government Should Invest in Materials Science
Materials science has historically delivered substantial economic benefits, but it is difficult to measure those benefits objectively. One reason it is difficult to measure those economic benefits is that economic growth is driven not just by the discovery of a new material, but by the technologies that emerge from it. Some modern industries are fundamentally founded on materials-science breakthroughs, without which modern life would not be the same. The semiconductor industry is a good example. Its value is estimated to have been worth more than $600 billion in 2023[21] and is projected by McKinsey to double in size by 2030.[22]
Another reason for the difficulty of measuring the economic impact of materials discoveries is that they often take considerable time to come to market. The first synthetic plastic was invented in 1869, but, at the time, it was only used in small-scale applications because of flaws in its initial synthesis (notably, its flammability). Only decades later, in 1907, with the invention of Bakelite,[23] did synthetic plastics come to be widely used. They were then refined and used primarily in industrial processes, only reaching widespread consumer use in the 1920s.[24]
Similar stories abound in materials science. Velcro, pioneered in France in the 1940s, had only modest usage until the 1960s when it was prominently used by NASA for the Apollo program. It was not until the 1970s that Velcro became widely used in consumer goods.[25] Lithium cobalt oxide, another example, was first synthesized in 1958,[26] but it would not be until the 1970s that researchers realized its potential as a cathode in a lithium-ion battery.[27] And it would take three more decades until lithium-ion batteries became common in daily life. The lithium-ion battery industry today is estimated at approximately $54 billion.[28]
Other times, materials are invented, used, fall out of favor, and then rediscovered. The glass materials company Corning developed Chemcor glass—a chemically strengthened variant of glass—in the 1960s, and it was used until the 1990s in industrial settings and specialty applications like racecars. Its usage largely stopped in the 1990s until, a bit more than a decade later, Apple would use the glass for the display covers on their nascent iPhone. Marketed today as Gorilla Glass, the material has been used on billions of mobile devices worldwide.[29]
Materials science discoveries sit on a wide spectrum of novelty and impact. Many discoveries are relatively simple iterations within existing classes of material. But even incremental improvements can have an outsized impact. For example, large numbers of iterative developments in aluminosilicate zeolites led to the synthesis of ZSM-5, which eventually became widely used in a broad range of industrial applications, most notably breaking down large hydrocarbon molecules, also known as “cracking.”[30] Other discoveries are serendipitous breakthroughs, where researchers are looking for something entirely different and stumble upon a truly novel material. TEFLON, for example, was discovered by accident when researchers at DuPont were investigating chlorofluorocarbon refrigerant gases.[31]
A common throughline in all these examples is the significant time it takes for novel materials to diffuse throughout industry and into broad-based usage. It takes time for firms to discover the uses of newly discovered materials, and it takes time to learn to mass manufacture new materials, re-engineer industrial processes to get them into products, and more. Ben Reinhardt has suggested that the time for transformative new materials to make it from the lab to widespread usage is approximately 50 years and that this pattern is “shockingly consistent.”[32] The economist Matt Clancy has argued that the benefits of basic research—going from lab experiments to a commercialized product—often take approximately 20 years, on average.[33] This significant time delay underscores the usefulness of public funding for materials science research; private sector-funded research often requires a much shorter time horizon to commercialization.
Fundamentally, much of materials science research is basic research and development. Economists have consistently found that research and development yield high returns over time. A 2023 study by the Federal Reserve Bank of Dallas found that government-funded, nondefense research and development yielded a return of between 150 percent and 300 percent during the postwar period.[34]
However, not all materials science research is “basic” research and development. Some, like innovations in battery chemistry or solar panel materials, can be commercialized more readily. Still, as we have seen, many novel materials do take considerable time to reach the level of mass manufacturability and commercialization required to generate a substantial consumer surplus.
Indeed, it is because of the long timelines to commercialization that many areas of materials science are under-invested in by the private sector. Academic, philanthropic, and government support is often required to fund this basic research in the absence of a private sector outlier, such as Bell Labs, whose decades of foundational research in a variety of fields (including materials science) was enabled by the monopoly on phone service granted to AT&T by the federal government.
Thus, we have strong evidence that materials science innovations can deliver meaningful economic growth, that government investment in research and development (which can include materials science) often yields high returns, and that the private sector is likely to under-invest in core aspects of materials science—particularly those without near-term opportunities for commercialization.
An Agenda for Accelerated Materials Discovery
To advance the pace and quality of materials discovery using AI, federal policymakers should pursue the following three objectives:
- Build cross-departmental materials science datasets and data infrastructure
- Partner with the private sector to develop materials science foundation models or, where sensitive data is being employed, build such models within government
- Create a competitive bidding process or “grand challenge” for private firms to offer proposals on the creation of robotic materials science labs (also referred to as “self-driving labs”)
More detail on each objective follows, and proposed budgets are included at the end of this section.
Build materials science datasets
Any AI model is fundamentally limited by the quality of data on which it is trained. Indeed, one way to think of AI models is as a “compression” of their training data.[35] A neural network’s “intelligence” is derived from being tasked with learning a training dataset that contains more discrete facts than can be stored. To “learn” the dataset, the neural network needs to identify “shortcuts”—patterns.
Current datasets for materials science are small in comparison to those used for training language models and even biological foundation models. Meta’s current frontier language model was trained on 15 trillion tokens or about 11 trillion words.[36] A recent DNA model was trained on 300 billion tokens—single nucleotides—in this case,[37] and the largest publicly known protein language model was trained on 771 billion tokens.[38] By contrast, the largest single publicly available dataset for inorganic materials science contains 110 million data points.[39], [40]
The federal government houses some of the richest materials science datasets in the country, if not the world. The Materials Project, one of the largest and most widely used materials science datasets, is supported by the Department of Energy, the National Science Foundation (NSF), as well as Lawrence Livermore and Argonne National Labs (themselves DOE user facilities).[41]
Independent from the Materials Project, DOE National Labs, particularly Argonne, Lawrence Livermore, Los Alamos, and Oak Ridge, house vast repositories of materials science data. For example, Los Alamos’s Neutron Science Center, Oak Ridge’s High Flux Isotope Reactor, and Spallation Neutron Source are among the most powerful neutron scattering facilities in the world and can be used to generate detailed atomic structure data for a wide range of materials.[42] The DOE’s Basic Energy Sciences Program funds hundreds of materials science experiments and other research across the country.[43] All of these experiments and facilities are sources of valuable data that can, at least in principle, be used for training foundation models.
Outside of DOE, the National Institute of Standards and Technology (NIST) is another source of vast materials science training data. NIST’s Materials Measurement Laboratory maintains reference databases of material properties.[44] NIST contributes to the multi-agency Materials Genome Initiative with extensive materials informatics databases, as well as standards for ensuring the quality and interoperability of materials datasets.[45]
The NSF supports research in materials science, as in most other domains of science. The NSF also funds facilities that collect ample materials science data, such as the National High Magnetic Field Laboratory at Florida State University.[46] Numerous other agencies, including the National Aeronautics and Space Administration (NASA), the National Nuclear Security Administration (NNSA), and US Geological Survey (USGS), and the Department of Defense, among others, also collect and maintain potentially valuable materials science data.
Unfortunately, there have been barriers to using this government data to train neural networks due to the fact that the datasets are housed in different agencies in different formats with different levels of quality and quantity, and often with terms of access and use that prohibit interagency sharing. Many datasets of material characteristics are maintained by NIST, for example, might be useful for pre-AI scientific and industrial applications, but are not nearly large enough to support AI model training.[47]
Even within a single agency, decentralized storage of data, outdated data infrastructure, and onerous data access and retention policies can make it challenging to use scientific datasets to their full potential. A 2023 DOE report that details the department’s challenges with data notes, for example, that DOE facilities produce so much data that they “are forced to roll-off [delete] data” due to a lack of sufficient storage capacity. The report goes on to describe the DOE’s “fragmented” data infrastructure and access policies, observing that they “lead to repetition of effort and dilution of capabilities that weaken the return on DOE’s investment in data production and storage.” Unsurprisingly, the analysis further observes that “in almost all cases, the requirements. . . of large-scale AI model training are not contemplated.”[48]
These challenges were, in theory, among those meant to be addressed by the Materials Genome Initiative (MGI). Created in 2011 by President Obama, the broad aim of the MGI was to dramatically reduce the time it takes to bring new materials from the lab to the market and to simultaneously reduce the cost of doing so.[49] One of the primary ways the initiative planned to accomplish these goals was to create “data and interoperability standards.” Numerous productive advancements, such as the NIST Materials Data Repository, were driven at least in part by the MGI. As a multi-agency initiative, funding and operational support was spread across approximately a dozen different federal departments.
Unfortunately, so, too, was leadership. And without centralized leadership, the MGI has struggled to accomplish some of its goals—particularly those requiring cross-agency collaboration and information sharing. The MGI’s 2021 Strategic Plan, which surveys the MGI’s first decade, notes the initiative’s success at generating new materials science datasets, but acknowledges that “there is still a great deal of work to be done to achieve the desired levels of integration and utility for the materials R&D enterprise.”[50]
A variety of reports and assessments on the MGI have made constructive recommendations for improving the state of data infrastructure.[51] Among these are ensuring that the NSF mandates that researchers adhere to FAIR (findable, accessible, interoperable, reusable)[52] guidelines for data management whenever possible, and that grant recipients complete data management plans to document the steps they will take to adhere to FAIR standards.
Other research has argued that even deeper changes to the practices and incentives of the scientific enterprise are required for leveraging AI in materials science. One paper, for instance, points to the need for a “transformation of scientific data governance toward AI” involving “researchers, funding institutions, publishing bodies, research equipment suppliers, data management platforms, and standardization organizations” whose “sustained attention and collaborative efforts are essential for achieving this transformation." The authors suggest creating incentives to recognize datasets as valuable contributions to the academic field, compared to the current incentive system that primarily favors papers and publication-worthy results. They also suggest making citation metrics for shared datasets and creating other academic impact measures for data contributions.[53]
A key recommendation to increase the usability of government datasets is that the President’s Office of Science and Technology Policy designate a formal individual or office to ensure the implementation of these data-related efforts across the federal government. Ideas for how to increase access to usable datasets are well-circulated, however, they are difficult to execute and fund. In other research on this topic, I estimate the cost of building a unified and appropriately secure data infrastructure for the DOE at approximately $100 million.[54] If funded and developed successfully, this shared data infrastructure could be the foundation for sharing materials data from other agencies and government-funded academic research.
Creating materials science foundation models
Just as materials science training data are small compared to data in other areas of AI, materials science foundation models themselves are often far smaller than those in domains like natural language processing or biology. Frontier language models range from thirty billion to over one trillion parameters.[55] The DNA model Evo is seven billion parameters,[56] and the protein language model ESM3 is ninety-eight billion parameters.[57]
Meanwhile, the top-performing models on MatBench, a benchmarking website for materials science AI models, are, at the time of writing, between hundreds of thousands or tens of millions of parameters. The best-performing model, from Meta, is a thirty-one million parameter transformer, using the same basic architecture that is used by the multi-billion parameter models mentioned in the preceding paragraph.
The difference in parameter count can be misleading. Materials science models can be smaller than models used in, for example, natural language processing because materials science data is generally structured and lower dimensional, and the predictions the models are trained to make are comparatively simpler (predicting the energy of a crystal, say, versus predicting thousands of words of a sequence of text).
Yet, if the past decade of the deep learning revolution has taught us anything, it is that the larger the scale—model size, training data, and computational power used to train models—the more remarkable results deep learning can deliver.[58] The relationships between these variables—and their tendency to deliver more performant neural networks when increased in tandem—have been referred to as “scaling laws.” While not laws of nature, scaling laws have been observed across a wide range of data modalities, including in scientific data. This implies there is substantial low-hanging fruit to be gained simply from increasing the size of materials science models, so long as more training data is available.
While the training of frontier language models is computationally expensive and has required the largest amalgamation of computing resources ever assembled, materials science models can likely be scaled up from their current frontier with far less computing power. GPT-2, a 124 million parameter transformer from 2019, can today be trained for approximately $20 in around 90 minutes using cloud computing services.[59]
Current materials science models from large technology firms, such as the Microsoft MatterGen and Google DeepMind’s GNoME, were produced in collaboration with US national labs (Lawrence Livermore in the case of GNoME, and Pacific Northwest National Lab in the case of Microsoft). Continued collaboration with AI companies on next-generation materials science models is advisable for national labs because doing so allows the government to bring the best possible private-sector expertise to bear and to maximize the efficiency of public spending by having private firms bear at least some of the cost of model training.
Broadly speaking, materials science-based models fall into two large categories. Some models can predict a compound’s structure based on desired characteristics (thermal performance, strength, flexibility, etc.)—these are often called “inverse design” models. Others predict various characteristics of a given input compound. Both types of models are useful, and they can both be used in combination with more traditional and computationally expensive simulation methods such as density functional theory (as discussed above in the example of the GNoME paper).
In addition to being used alongside more traditional computational modeling methods, materials science models can also be combined with other kinds of AI models. For example, a recent paper from DeepMind described the combination of an AI diffusion model (a specific kind of model, also used in the latest version of AlphaFold) used to generate crystal structures, a graph neural network used to make predictions about those generated structures, and a language model that allows natural language input. Together, the system allows users to input desired material properties in natural language and generate (potentially) workable crystal structures along with predictions about that crystal’s real-world characteristics, such as its formation energy.[60]
Just as with materials data, it is better for the scientific enterprise if these models are broadly available to the public or, at least, to the academic community. Indeed, materials science models should be designed and trained with public release in mind. The existence of a new tool does not, in itself, accomplish much: It is people using tools that creates utility and new knowledge. Viewed in this light, public disclosure is a benefit to scientific discovery, not a cost. Thus, materials science foundation models made by the government should be made available to at least the scientific community, if not the general public.
There may be examples of AI applications that require sensitive training data or whose output may not be useful to a broad range of academic or corporate users. For example, the DOE houses an enormous amount of data related to nuclear weapons, primarily through the NNSA, that is both highly sensitive and only useful to DOE itself and a small range of other actors. Models using data of this kind will have to be trained in-house if they are deemed desirable. This underscores the importance of ensuring a shared data infrastructure—built with AI model training in mind—throughout the federal government, with different security parameters for data of differing sensitivity levels.
Constructing self-driving labs
The predictions of any AI foundation model for science are just that: predictions. To be useful, they must be tested. And even if models become so good that their predictions are nearly perfect or otherwise highly reliable, there will still be the nontrivial process of learning how to produce the compound, even in artisanal batches. Just as with any complex production process, or even cooking just a simple meal, the specific sequence and manner in which steps are taken greatly affects the final product. Learning how to synthesize a novel material is itself an exceptionally high-dimensional problem, requiring many iterations of experiments to complete.
Turning an AI-predicted material into a real-world experiment can be split into two broad steps: synthesis and characterization. Synthesis is the process of making an (often) artisanal amount of a desired novel material. Characterization is assessing the relevant properties of that material, often comparing them against predictions (in our case, the predictions of an AI model).
Synthesis involves developing a plan for producing the compound, testing that plan, and making iterative adjustments to the process based on real-world results. The process inherently involves trial and error, often with small iterations to targeted portions of highly repetitive steps. Characterization, at its heart, is about measurement, whether measuring structure (with X-ray diffraction or microscopy), chemical composition (using various forms of spectroscopy), thermal properties like melting point or heat capacity (using differential scanning calorimetry), and electrical properties like conductivity (using a four-point probe).
Both of these broad steps are susceptible to automation, as has been pointed out in numerous papers.[61] Materials science labs that automate these steps are often referred to as “self-driving labs.” While the idea of a self-driving lab is not new, recent advancements in robotics hardware, AI-based computer vision software, and even AI agents to orchestrate scientific experiments have brought this vision far closer to reality.
In just the past few years, self-driving labs have crossed the threshold of “proof of concept” and have begun to yield real results. Some small-scale self-driving labs have already been built and have generated results published in scientific research. Researchers recently used autonomous software and hardware to discover, test, and produce novel solid state laser materials that are best-in-class for certain use cases.[62] The A-Lab, a small-scale self-driving lab at Lawrence Livermore National Lab, successfully synthesized AI-generated materials.[63] The University of Toronto’s Matter Lab has produced both software[64] and hardware[65] platforms for automated materials discovery and synthesis.
Even more intriguingly, large, generalist, multimodal AI models like ChatGPT are reaching the level of expertise where they, too, can play a key role in orchestrating experiments. Current frontier models like OpenAI’s GPT-4o and o1-preview and Anthropic’s Claude 3.5 Sonnet are approaching and sometimes exceeding human expert performance at a variety of science, technology, engineering, and mathematics benchmarks.[66] For example, 3.5 Sonnet scores 65 percent on the PhD-level GPQA science benchmark, just behind human domain expert performance of 69 percent; OpenAI’s o1 scores 78 percent. And new model features, such as the ability to fully operate a computer as a human would (recently introduced by Anthropic),[67] mean that, increasingly, the most advanced generalist AI models will be able to serve as “automated graduate students.”
While these generalist models may not be able to autonomously ideate and execute experiments, they can be experimented with as hour-to-hour and day-to-day overseers of experiments conducted in self-driving labs. In this workflow, human scientists would provide the experimental goals and the overarching framework for, to give an example, synthesizing a specific compound whose structure was predicted by another AI model. A self-driving lab could begin carrying out initial synthesis steps and provide data to the automated graduate student. The automated graduate student could then suggest appropriate iterations to the experiments, while holding, for example, the entire relevant academic literature in its active attention window simultaneously. Many current models have sufficient context windows to hold entire bodies of academic literature in their attention at one time, as well as the visual understanding to interpret charts and graphs.[68]
Meanwhile, robotics is becoming more sophisticated—often driven by advancements adjacent to those seen in generalist AI models. Recent systems from Google DeepMind[69] and the startup Physical Intelligence,[70] for example, combine internet-scale image and text data (vision-language models) with learned robot actions to train generalist robotic models. These models are generalists, not just because they can (in principle) operate in a wide range of environments to do a variety of tasks, but because they embody a range of different robotic hardware with intelligence.
In all cases, there are challenges to building self-driving labs beyond automation per se. Materials characterization relies on a range of measurement tools such as scanning electron, transmission electron, and atomic force microscopes, X-ray photoelectron spectroscopes, and diffraction tools. Many of these tools are exclusively designed for artisanal-scale materials science and cannot be easily scaled to industrial-scale materials characterization. They are designed to analyze one or a handful of samples at a time and thus are not designed for parallel processing of tens, hundreds, or even thousands of samples. One of the bottlenecks to scaling materials science may well be the creation of new metrology tools.
Given the number of unanswered questions, the ideal way to pursue the construction of a materials science self-driving lab is to allow the private sector to offer a diverse range of ideas in a competition for a federal grant. Charles Yang has suggested a “Grand Challenge” model, akin to the Defense Advanced Research Projects Agency (DARPA) autonomous vehicle grand challenges of the early 2000s.[71] This is a model that has yielded results in the past. And because much of the expertise in robotics, AI, and materials science itself lies outside of the federal government, it is optimal to take an approach that allows for private firms to competitively bid, or to collaborate, for the opportunity to construct government-funded labs.
Another approach would be a more traditional public-private partnership. This could be facilitated using the DOE’s new Foundation for Energy Security and Innovation, a foundation that simplifies the process of creating partnerships between private firms and government agencies and creating government fellowships for private sector talent.[72] DOE would be a natural locus for this partnership because of its central role in federally funded materials science research and because it is a hub for materials science datasets. There are already small-scale self-driving labs at both Argonne and Lawrence Livermore, and these facilities could be logical starting points for larger initiatives. In addition, the DOE already has experience managing scientific resources that are used by researchers nationwide.
Estimated budget
Table 1 shows an estimated budget for achieving all three goals described above. This funding could be apportioned at once or over a period of years. Furthermore, some of the individual budget items could likely be shared between the public and private sectors.
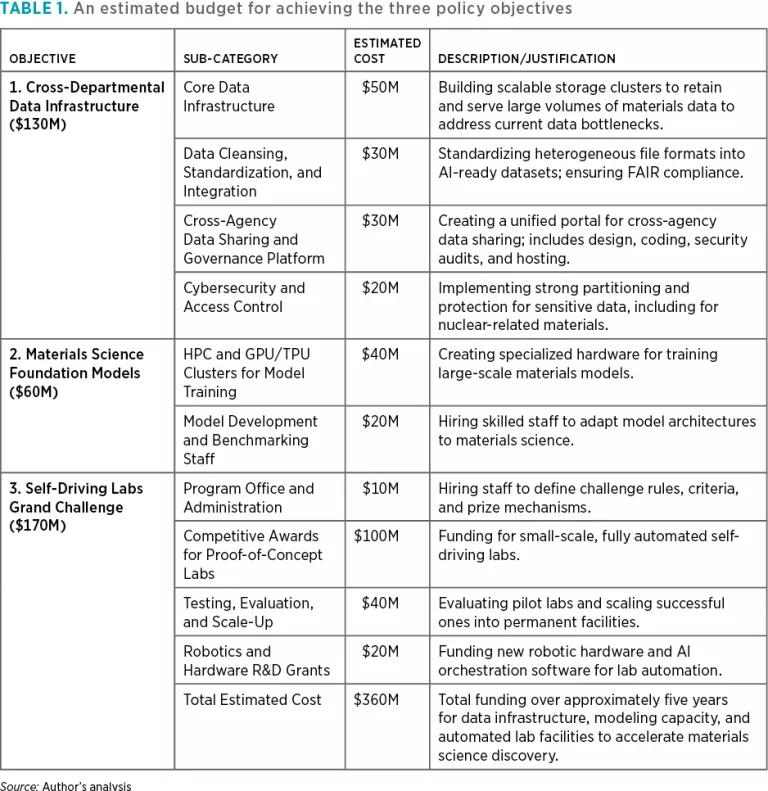
Conclusion
This paper presents a vision for the transition of science from the artisanal scale to the industrial. If executed successfully, the plan described here could enable orders of magnitude more materials science experiments to be conducted annually at a far lower cost. Some of the results of that are predictable: more efficient industrial processes, better batteries, more durable consumer goods. Other results seem more distant possibilities from our vantage point today: better magnets to make commercial nuclear fusion a reality, or superior ceramics to enable cheaper spacecraft and hypersonic missiles. Even others seem truly outlandish: room-temperature superconductors, on their own, could represent an innovation as significant as steel, internal combustion, or electricity. But there was a time when every radical new invention seemed impossible or outlandish; the history of science and technology is making impossible things feasible, and eventually, commonplace.
As we have seen, many materials science innovations are the result of thousands of iterative adjustments to existing classes of materials. Others are the result of serendipity. We cannot, a priori, know the process by which the next big discovery will come, so the best way forward is to get far more shots on goal. The more we try, the higher our likelihood of success. And the plan presented here has exactly this objective at its foundation.
This report touches on themes that are likely to be seen as AI transforms other domains of science, and even other areas of economic life altogether. AI models—whether narrow models trained to predict the energy of a crystal or generalist models meant to mimic human intelligence—can produce insights and ideas. But they cannot make those things into reality. And their predictions will only be as good as the data they are fed.
Without systematic changes to the way we conduct materials science, new ideas will be held back by the bottlenecks of the past. Without new approaches to sharing data and information, AI models in the US will be inherently impoverished. Inevitably, other countries will change their institutions to maximize the value of AI, and if the US does not keep up, it will be left behind. The US today leads in innovation at the frontier of AI, but those innovations mean little if they are not used as widely and creatively as possible. There is nothing easy about broad diffusion of a powerful general-purpose technology that fundamentally challenges long-held assumptions. Yet this is the task the United States faces. Whether we seize the opportunity or shrink before it is our choice to make.
About the Author
Dean W. Ball is a research fellow at the Mercatus Center at George Mason University and author of the Substack Hyperdimensional. His work focuses on AI, emerging technologies, and the future of governance. Previously, he was senior program manager for the Hoover Institution’s State and Local Governance Initiative.
Acknowledgments
The author wishes to express his gratitude to the RAND Corporation and Fathom for their support of this work. The views expressed in this document are those of the author and do not necessarily reflect the opinions of RAND or Fathom.
Notes
[1] David S. Landes, Revolution in Time: Clocks and the Making of the Modern World (Belknap Press, 2000).
[2] Joel Mokyr, The Lever of Riches (Oxford University Press, 1992).
[3] William D. Callister and David G. Rethwisch, Materials Science and Engineering: An Introduction, 8th ed. (Wiley, 2010), 3.
[4] Amil Merchant and Ekin Dogus Cubuk, “Millions of New Materials Discovered with Deep Learning,” Google DeepMind, November 29, 2023, https://deepmind.google/discover/blog/millions-of-new-materials-discove….
[5] Andrew W. Senior et al., “Improved Protein Structure Prediction Using Potentials from Deep Learning,” Nature 577 (January 15, 2020): 706–10.
[6] John Jumper et al., “Highly Accurate Protein Structure Prediction with AlphaFold,” Nature 596 (July 15, 2021): 583–89.
[7] Josh Abramson et al., “Accurate Structure Prediction of Biomolecular Interactions with AlphaFold 3,” Nature 630 (May 8, 2024): 493–500.
[8] Eric Nguyen et al., “Sequence Modeling and Design from Molecular to Genome Scale with Evo,” Science 386, no. 6723 (2024).
[9] Adly Templeton et al., “Scaling Monosemanticity: Extracting Interpretable Features from Claude 3 Sonnet,” Transformer Circuits Thread, May 21, 2024.
[10] Ibrahim Alabdulmohsin, Vinh Tran, Mostafa Dehghani, “Fractal Patterns May Illuminate Success of Next-Token Prediction,” paper presented at NeurIPS 2024, Vancouver, December 2024.
[11] G.E. Hinton, J.L. McClelland, and D.E. Rumelhart, “Distributed Representations” in Parallel Distributed Processing: Explorations in the Microstructure of Cognition, vol. 1: Foundations, eds. James McClell and David Rumelhard (MIT Press, 1986).
[12] Elana Simon and James Zou, “InterpLM: Discovering Interpretable Features in Protein Language Models via Sparse Autoencoders,” bioRxiv (November 13, 2024).
[13] Thomas Hayes et al., “Simulating 500 Million Years of Evolution with a Language Model,” Science 387, no. 6736 (January 16, 2025).
[14] Thomas C. Terwilliger et al., “AlphaFold Predictions Are Valuable Hypotheses and Accelerate but Do Not Replace Experimental Structure Determination,” Nature Methods 21 (November 30, 2023): 110–116.
[15] Claudio Zeni et al., “MatterGen: A Generative Model for Inorganic Materials Design,” arXiv (January 24, 2024).
[16] See, for example, Jehad Abed et al., “Open Catalyst Experiments 2024 (OCx24): Bridging Experiments and Computational Models,” arXiv (November 2024) and “Open Catalyst Project,” Meta/Carnegie Mellon University, last accessed February 14, https://opencatalystproject.org.
[17] Edward O. Pyzer-Knapp et al., “Accelerating Materials Discovery Using Artificial Intelligence, High Performance Computing and Robotics,” npj Computational Materials 8, no. 84 (April 26, 2022).
[18] Amil Merchant et al., “Scaling Deep Learning for Materials Discovery,” Nature 624 (November 29, 2023): 80–85.
[19] It is important to note that, despite headlines replete with warnings about the tremendous power consumption of AI, in many scientific domains AI is in fact farmore power efficient than traditional computational methods for simulating scientific phenomena.
[20] Josh Leeman et al., “Challenges in High-Throughput Inorganic Materials Prediction and Autonomous Synthesis,” PRX Energy 3, no. 011002 (March 7, 2024).
[21] World Semiconductor Trade Statistics, “WSTS Semiconductor Market Forecast Fall 2024,” news release, December 3, 2024.
[22] Ondrej Burkacky, Julia Dragon, and Nikolaus Lehmann, “The Semiconductor Decade: A Trillion-Dollar Industry,” McKinsey and Company, April 2, 2022, https://www.mckinsey.com/industries/semiconductors/our-insights/the-semiconductor-decade-a-trillion-dollar-industry.
[23] Joel Mokyr, The Lever of Riches.
[24] Joris Mercelis, Beyond Bakelite: Leo Baekeland and the Business of Science and Invention (MIT Press, 2020).
[25] “Our Timeline of Innovation,” Velcro, last accessed February 15, 2025, https://www.velcro.com/original-thinking/our-timeline-of-innovation/.
[26] W.D. Johnston, R.R. Heikes, D. Sestrich, “The Preparation, Crystallography, and Magnetic Properties of the LixCo(1−x)O System,” Journal of Physics and Chemistry of Solids 7, no. 1 (1958).
[27] Anthony K. Cheetham, Ram Seshadri, and Fred Wudl, “Chemical Synthesis and Materials Discovery,” Nature Synthesis 1 (June 30, 2022): 514–20.
[28]“Lithium-ion Battery Market Size & Trends,” Grand View Research, last accessed February 15, 2025, https://www.grandviewresearch.com/industry-analysis/lithium-ion-battery-market.
[29] “Corning’s History of Chemically Strengthening Glass,” Corning, last accessed February 15, 2025, https://www.corning.com/worldwide/en/innovation/materials-science/glass….
[30] T.F. Degnan, G.K. Chitnis, and P.H. Schipper, “History of ZSM-5 Fluid Catalytic Cracking Additive Development at Mobil,” Microporous and Mesoporous Materials 35–36 (April 2000).
[31] Degnan, Chitnis, and Schipper, “History of ZSM-5 Fluid.”
[32] Benjamin Reinhardt, “Getting Materials out of the Lab,” Works in Progress, no. 15 (May 2024).
[33] Matt Clancy, “How Long Does It Take to Go from Science to Technology?” New Things Under the Sun, (August 2021).
[34] Andrew J. Fieldhouse and Karel Mertens, “The Returns to Government R&D: Evidence from US Appropriations Shocks,” Federal Reserve Bank of Dallas, working paper 2305 (2023).
[35] Grégoire Delétang et al., “Language Modeling Is Compression,” arXiv (September 19, 2023).
[36] Aaron Grattafiori et al., “The Llama 3 Herd of Models,” arXiv (July 31, 2024).
[37] Grattafiori et al., “The Llama 3 Herd of Models,” 8.
[38] Grattafiori et al., “The Llama 3 Herd of Models,” 13.
[39] Luis Barroso-Luque et al. “Open Materials 2024 (OMat24) Inorganic Materials Dataset and Models,” arXiv, (last accessed February 15, 2025).
[40] Please note that this is a bit of an apples-to-oranges comparison. The number of data points in the materials science dataset referenced would likely be lower than the number of tokens used to train a transformer-based AI model. Also note that tokens as a measure of training dataset size are most commonly used for language (or language-like) data modalities; the “token” as a concept may not be used in the kinds of models used for materials science. With those points said, the rough order of magnitudes here is the core point: current materials science training data is smaller than in other fields.
[41] “Partners and Support,” The Materials Project, last accessed February 15, 2025, https://next-gen.materialsproject.org/about/partners.
[42] “High Flux Isotope Reactor,” Oak Ridge National Laboratory, last accessed February 15, 2025, https://neutrons.ornl.gov/hfir; “Spallation Neutron Source,” Oak Ridge National Laboratory, last accessed February 15, 2025, https://neutrons.ornl.gov/sns; and “Los Alamos Neutron Science Center,” Los Alamos National Science Center, last accessed February 15, 2025, https://lansce.lanl.gov.
[43] “Materials Science and Engineering Division,” US Department of Energy, last accessed February 15, 2025, https://science.osti.gov/bes/mse.
[44] “Office of Reference Materials,” NIST, last accessed February 15, 2025, https://www.nist.gov/mml/orm.
[45] “The Materials Genome Initiative at NIST,” NIST, last accessed February 15, 2025, https://www.nist.gov/pao/materials-genome-initiative-nist.
[46] National High Magnetic Field Laboratory, last accessed February 15, 2025, https://nationalmaglab.org.
[47] From an interview with anonymous materials science startup executive.
[48] Jonathan Carter et al., Advanced Research Directions on AI for Science, Energy, and Security: Report on Summer 2022 Workshops (Argonne National Laboratory, May 2023).
[49] “Goals of the Materials Genome Initiative,” The White House/President Obama, last accessed February 15, 2025, https://obamawhitehouse.archives.gov/mgi/goals.
[50] National Science and Technology Council Subcommittee on the Materials Genome Initiative, Materials Genome Initiative Strategic Plan (Committee on Technology, 2021).
[51] See, for example: “Building a Materials Data Infrastructure: Opening New Pathways to Discovery and Innovation in Science and Engineering,” The Minerals and Metals Society (2017) and “NSF Efforts to Achieve the Nation’s Vision for the Materials Genome Initiative: Designing Materials to Revolution and Engineer Our Future (DMREF),” National Academy of Sciences, Engineering, and Medicine (2023).
[52] Mark D. Wilkinson et al., “The FAIR Guiding Principles for Scientific Data Management and Stewardship,” Scientific Data 3, no. 1 (March 15, 2016).
[53] Yongchao Lu et al., “Unleashing the Power of AI in Science-key Considerations for Materials Data Preparation,” Scientific Data 11, no. 1 (September 27, 2024).
[54] Dean W. Ball, “Acceleration Materials Science with AI and Robotics,” Federation of American Scientists, 2024, https://fas.org/publication/accelerating-materials-science-with-ai-and-robotics/.
[55] Many proprietary language model providers, such as OpenAI and Anthropic, no longer disclose parameter counts for their frontier models, but Meta’s Llama 3 series ranges from 8 to 405 billion parameters, and the original version of GPT-4 from March 2023 was rumored to have been 1.8 trillion parameters.
[56] Ball, “Acceleration Materials Science with AI and Robotics,” 8.
[57] Ball, “Acceleration Materials Science with AI and Robotics,” 13.
[58] Jared Kaplan et al., “Scaling Laws for Neural Language Models,” arXiv (January 23, 2020).
[59] Andrej Karpathy, “Let’s Reproduce GPT-2 (124M),” June 9, 2024, https://www.youtube.com/watch?v=l8pRSuU81PU.
[60] Sherry Yang et al., “Generative Hierarchical Materials Search,” arXiv, (September 10, 2024).
[61] See, for example, Edward O. Pyzer-Knapp et al., “Accelerating Materials Discovery Using Artificial Intelligence, High Performance Computing and Robotics,” Npj Computational Materials 8, no. 1 (April 26, 2022); Milad Abolhasani and Eugenia Kumacheva, “The Rise of Self-driving Labs in Chemical and Materials Sciences,” Nature Synthesis 2, no. 6 (January 30, 2023): 483–92; Accelerated Materials Experimentation Enabled by the Autonomous Materials Innovation Infrastructure: A Workshop Report, Materials Genome Initiative Autonomous Materials Innovation Infrastructure Interagency Working Group (National Science Foundation, June 2024); Ball, “Acceleration Materials Science with AI and Robotics;” and Charles Yang, “Automating Scientific Discovery: A Research Agenda For Advancing Self-Driving Labs,” Federation of American Scientists (January 31, 2024).
[62] Felix Strieth-Kalthoff et al., “Delocalized, Asynchronous, Closed-loop Discovery of Organic Laser Emitters,” Science 384, no. 6697 (May 16, 2024).
[63] Nathan J. Szymanski et al., “An Autonomous Laboratory for the Accelerated Synthesis of Novel Materials,” Nature 624, no. 7990 (November 29, 2023): 86–91.
[64] Shi Xuan Leong et al., “Automated Electrosynthesis Reaction Mining with Multimodal Large Language Models,” Chemical Science 15, no. 17881 (2024).
[65] Sergio Pablo-García et al., “An Affordable Platform for Automated Synthesis and Electrochemical Characterization,” ChemRxiv (2024).
[66] “Introducing Computer Use, a New Claude 3.5 Sonnet, and Claude 3.5 Haiku,” Anthropic.com, October 22, 2024, https://www.anthropic.com/news/3-5-models-and-computer-use; “Hello GPT-4o,” Openai.com, May 13, 2024, https://openai.com/index/hello-gpt-4o/; “Learning to Reason with LLMs,” Openai.com, September 12, 2024, https://openai.com/index/learning-to-reason-with-llms/.
[67] “Developing a Computer Use Model,” Anthropic.com, October 22, 2024, https://www.anthropic.com/news/developing-computer-use.
[68] Frontier context lengths vary between 128,000 and several million tokens (between the length of a novel or the length of roughly 20 novels).
[69] Abby O’Neill et al., “Open-X Embodiment: Robotic Learning Datasets and RT-X Models,” arXiv (October 2023).
[70] Kevin Black et al., “π0: A Vision-Language-Action Flow Model for General Robot Control,” Physical Intelligence (October 2024).
[71] See Yang, “Automating Scientific Discovery;” and Charles Yang and Hideki Tomoshige, “Self-Driving Labs: AI and Robotics Accelerating Materials Innovation,” Center for Strategic and International Studies (January 5, 2024).
[72] “Foundation for Energy Security and Innovation (FESI),” US Department of Technology, last accessed February 15, 2025, https://www.energy.gov/technologytransitions/foundation-energy-security-and-innovation-fesi.